The Pearson correlation coefficient (denoted as “r”) is widely used in statistics to measure the strength and direction of linear relationships between variables, ranging from -1 (perfect negative linear correlation) to +1 (perfect positive linear correlation). A Pearson correlation coefficient of 0 typically implies the absence of a linear relationship between variables. However, the term “linear” holds a key distinction that becomes evident when we explore an example where the Pearson Correlation Coefficient equals 0.
The Significance of Linearity:
The term “linear” is pivotal in understanding the limitations of the Pearson Correlation Coefficient. To illustrate this, let’s consider an example where the Pearson Correlation Coefficient is calculated as 0. Despite this apparent lack of correlation, there exists a meaningful relationship between the variables.
Example:
Imagine a scenario where the relationship between two variables, X and Y, is defined as follows: Y = X^2.
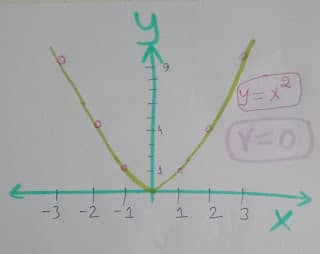
X -3 -2 -1 +1 +2 +3
Y +9 +4 +1 +1 +4 +9
If we compute the Pearson Correlation Coefficient for this dataset, it will be 0, signifying no linear
correlation. However, it’s essential to recognize that Y is indeed dependent on X through a non-linear
relationship (Y = X^2). This particular relationship forms a curve, as visually depicted above, and the
Pearson Correlation Coefficient is ill-suited to capture such non-linear associations.
Linear vs. Non-linear Relationships:

Linear relationships are characterized by straight-line patterns, which are not observed in the example above. The Pearson Correlation Coefficient excels at quantifying the strength and direction of linear correlations but falls short when dealing with non-linear relationships.
Addressing Non-linear Relationships:
When confronted with non-linear relationships, its crucial to explore alternative correlation measures that are better suited to capture such associations. Some options include:
Spearman’s rank correlation coefficient
Kendall’s rank correlation coefficient
In certain cases, applying transformations to the variables, such as logarithmic transformations, can be useful in making non-linear relationships more amenable to analysis. For further insights on handling non-linear relationships, you may refer to the provided blog resource.
Conclusions
In conclusion, understanding the nuances of linear and non-linear relationships is vital in interpreting
the significance of correlation coefficients like Pearson’s r. When dealing with non-linear associations, alternative methods and transformations should be considered to gain a more comprehensive understanding of the data.